Torrent details for:
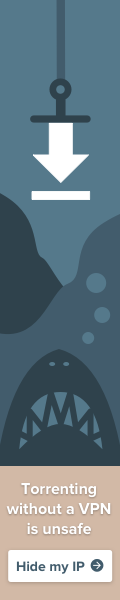
Roy K. Materials Informatics I. Methods 2025
Textbook in PDF format
This contributed volume explores the integration of machine learning and cheminformatics within materials science, focusing on predictive modeling techniques. It begins with foundational concepts in materials informatics and cheminformatics, emphasizing quantitative structure-property relationships (QSPR). The volume then presents various methods and tools, including advanced QSPR models, quantitative read-across structure-property relationship (q-RASPR) models, optimization strategies with minimal data, and in silico studies using different descriptors. Additionally, it explores machine learning algorithms and their applications in materials science, alongside innovative modeling approaches for quantum-theoretic properties. Overall, the book serves as a comprehensive resource for understanding and applying machine learning in the study and development of advanced materials and is a useful tool for students, researchers and professionals working in these areas.
Introduction
Introduction to Materials Informatics
Introduction to Cheminformatics for Predictive Modeling
Introduction to Machine Learning for Predictive Modeling of Organic Materials
Quantitative Structure–Property Relationships (QSPR) for Materials Science
Methods and Tools
Quantitative Structure–Property Relationships (QSPR) and Machine Learning (ML) Models for Materials Science
Optimising Materials Properties with Minimal Data: Lessons from Vanadium Catalyst Modelling
In Silico QSPR Studies Based on CDFT and IT Descriptors
Applications of Quantitative Read-Across Structure–Property Relationship (q-RASPR) Modeling in the Field of Materials Science
Machine Learning Algorithms for Applications in Materials Science I
Machine Learning Algorithms for Applications in Materials Science II
Structure-Property Modeling of Quantum-Theoretic Properties of Benzenoid Hydrocarbons by Means of Connection-Related Graphical Descriptors
Machine Learning Tools and Web Services for Materials Science Modeling
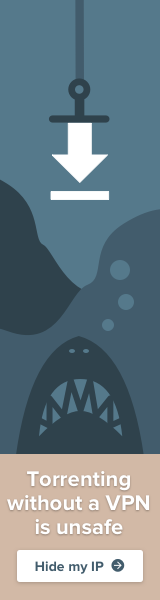